Navigating AI Challenges: Precision Tuning of Language Models for Business Success
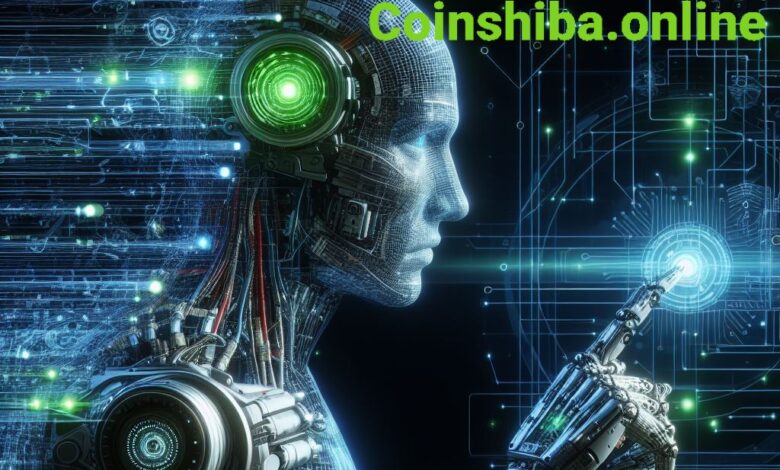
In the realm of Artificial Intelligence (AI), the emergence of Large Language Models (LLMs) promises companies intriguing prospects for enhancing decision-making processes, streamlining operations, and fostering groundbreaking innovation.
Prominent companies such as Zendesk, Slack, Goldman Sachs, GitHub, and Unilever have successfully harnessed LLMs to bolster customer support, improve coding processes, and efficiently handle customer inquiries. However, the Master of Business Administration (MBA), despite its strength, often falls short when confronted with the unique complexities of organizational contexts.
Challenges in Precisely Tuning AI Language Models
To overcome this challenge, companies have turned to refining Master of Business Administration (MBA) models by utilizing enterprise-specific information, a practice that yields highly tailored AI models. These precision-tuned models offer a bespoke intelligence experience, significantly enhancing organizational performance.
Nevertheless, venturing into AI model refinement poses immediate challenges for companies. This endeavor requires broad access to high-quality data, which is often a scarce resource for many organizations. Additionally, LLMs rely on publicly available internet information, leading to biases and a lack of diversity in the generated content.
Training precisely tuned models using user’s personal data raises significant privacy concerns, potentially resulting in regulatory violations. To overcome these challenges, organizations must proceed with caution, secure explicit consent for data usage, and ensure compliance with regional and international privacy standards, maintaining data integrity and safety throughout the data lifecycle.
Overcoming Data-Related Challenges in AI Precision Tuning
Precision-tuned AI models thrive on extensive and diverse datasets. However, many organizations face difficulties in obtaining necessary data, particularly in specialized fields. The problem exacerbates when available data is unorganized or of poor quality, hindering meaningful insights. Beyond quantity, the importance of data accuracy, representation, and diverse perspectives are critical considerations.
General AI models, including LLMs, largely reflect the broader internet, overlooking nuanced differences in specific communities or user groups. Consequently, these models often produce biased, culturally insensitive, or incomplete outputs, neglecting experiences and perspectives of specific societal groups.
To enrich these models, organizations must supplement them with data that genuinely represents societal diversity. This ensures that AI responses are comprehensive, unbiased, and culturally aware.
Embracing Data Collaboration Platforms
Fortunately, a glimmer of hope has emerged in the form of data collaboration platforms. These platforms provide a secure training space where high-quality, abundant data coexists with strict privacy compliance. They enable third parties to gain insights from personal data without tracing it back to the source, preserving data privacy and integrity.
Data collaboration platforms serve as a lifeline for organizations grappling with data scarcity. By facilitating collective fine-tuning of AI models without the need to share raw data, these platforms address challenges related to data quantity and quality.
Leadership in AI Business Transformation
To harness the full potential of precision-tuned models, business leaders must consider several key steps:
Customization is Vital: Ready-made AI tools, while advanced, may lack the context and specific nuances of the enterprise. Customization is crucial to align AI models with unique requirements.
Diverse, High-Quality Datasets: Diverse and high-quality datasets are essential for accurate and unbiased AI responses. Collaborative efforts in data sharing can significantly enhance model performance and diversity.
Collaborate, Even with Competitors: Beyond partnerships with clients and partners, consider collaboration with competitors. Collective efforts can lead to innovations and efficiencies that benefit the entire industry.
READ MORE Addressing Ethical Concerns: Universities Expand AI Education to Combat Deepfakes and Disinformation"
Regular Model Adjustment: Data is susceptible to change, and AI models should be precisely tuned based on the latest information. Seek current, relevant data sources for effective AI problem-solving.
In summary, navigating the AI landscape involves overcoming challenges related to data, biases, and privacy. By embracing precision tuning, collaborating on data platforms, and ensuring ongoing model adjustments, businesses can unlock the full potential of AI for transformative success.
Important Notice: Disclaimer Regarding Financial Advice
The information presented in this article is intended solely for informational purposes and should not be considered as financial advice. Coinshiba.online disclaims any responsibility for investment decisions made by individuals relying on the information provided herein. It is highly recommended to consult with a qualified professional or financial advisor before making any investment decisions. Your financial well-being is crucial, and seeking expert guidance ensures that your investment choices align with your individual financial goals and risk tolerance.
One Comment